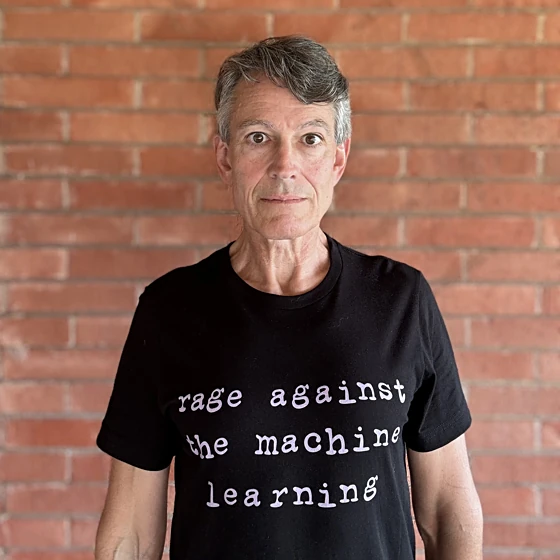
I finished a PhD in artificial intelligence in 1990.1 My academic papers from that era have been cited several thousand times. However, I left the field in 1993, and mainly ignored it until 2022.
One reason I stopped doing AI was a risk concern. Working in machine vision, I thought of a new approach to face recognition. That was considered an important unsolved problem at the time. It seemed to me, though, that face recognition was more likely to be used for oppressive surveillance than anything of net positive value; so I didn’t want to proceed.
Broadening the analysis, AI overall seemed likely to have net negative value in human terms—however interesting it was scientifically, and however potentially valuable financially. I still think that.
That wasn’t the only reason I left AI research. Vintage 1993 computers were absurdly slow, and also the field had come to an intellectual dead end. It was a good time to leave: both factors did mean that there was very little progress for two decades.
Symbolic AI conclusively failed in the late 1980s. Discouraging computational complexity results I proved in 1986 contributed to that.2
“Neural networks” generated a lot of hype in the late ’80s and early ’90s. (“Neural networks” are almost perfectly dissimilar to biological neural networks; the term mainly refers to “backprop,” the error backpropagation algorithm.) The hope was that they would overcome the limitations of symbolic AI, and provide a new way forward. I replicated several of the key “neural network” experimental results. I found that in each case the experimenters had fooled themselves, and their networks were not doing what they thought. So much for that.
In 2014, after ignoring AI for two decades, I learned about the 2012 AlexNet results. Backprop (rebranded as “deep learning”) performed startlingly well on an image classification benchmark. That got my attention, and I read a bunch of papers to figure out what was going on. I concluded that, once again, backprop had fooled researchers into thinking it was doing something it wasn’t. I summarize that analysis in “Massive parallelism and surface features.” Subsequent research by others showed that I was correct.
I paid a small amount of attention to AI out of the corner of my eye during 2015-2021, in case something else might happen. My impression was that the pattern was repeating. Backprop is a power tool for self-deception, and the dysfunctional epistemic culture of AI incentivizes spectacular demos rather than scientific understanding or engineering discipline. There were numerous heavily hyped results that I was pretty sure didn’t mean what they were said to.
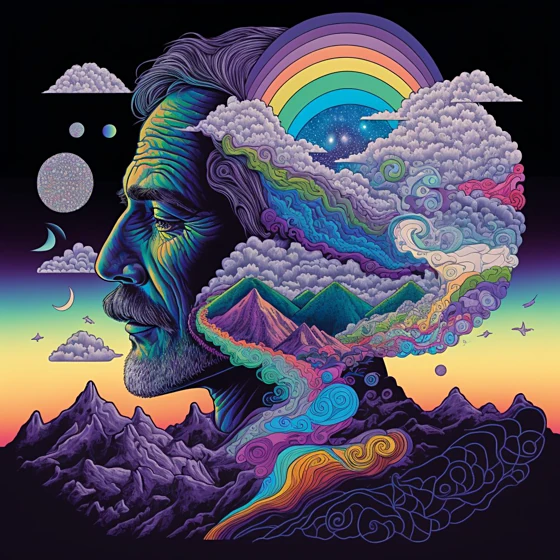
In mid-2022, I was startled by AI for the second time. Language models could be coaxed into producing what look like chains of reasoning:
Input: Trevor has wanted to see the mountain with all of the heads on it for a long time, so he finally drove out to see it. What is the capital of the state that is directly east of the state that Trevor is currently in?
Model Output: The mountain with all of the heads on it is Mount Rushmore. Mount Rushmore is in South Dakota. The state directly east of South Dakota is Minnesota. The capital of Minnesota is St. Paul. The answer is “St. Paul”.3
I wasn’t expecting that, and it spooked me. That reinvigorated my interest in artificial intelligence research. (I analyze this example in Are language models Scary.) It synergized with my concern with increasingly powerful malign effects of recommender AIs on culture, society, and individual psychology.
Together, those concerns led me to write Better without AI, a book that explains actions you can take to avert potentially apocalyptic effects of AI, and help bring about a future we would like better.
- 1.David Chapman, Vision, Instruction, and Action, MIT Press, 1991.
- 2.David Chapman, “Planning for conjunctive goals,” Artificial Intelligence 32:3, July 1987, pp. 333–377.
- 3.Chowdhery et al., “PaLM: Scaling Language Modeling with Pathways.”